Predictive Maintenance: A Mission Possible with Process Simulation
Simulation models enable engineering and planning departments to collaborate easily for making predictive maintenance a “mission possible”. Having your machine park within a virtual simulated environment, together with sales and/or production plans we can adequately stress the processes and evaluate which are the most prominent machine arrangements for each individual sale. Moreover, it can be further enhanced by adding service maintenance schedules, repair and/or resource availability criteria which will show us how production is affected by any of these parameters and their changes.
Making the right decisions is crucial for a lean production process. As much as this may sound like a cliché, it is yet very difficult to achieve in practice. These decisions are often based on previous experience, forecasts, and other factors, which naturally lead to subjective results. Not only may it be unclear why a particular outcome occurs but lead to production disruptions.
Bird Eyes View DES: Gaining data insights
During the research and development of industrial projects, a detailed simulation model of the given process is constructed. By testing multiple potential scenarios, information-driven designs are generated, showing precise sizing of the required equipment. This approach enables a comprehensive evaluation and budget planning of the analyzed system’s capacity and potential future improvements.
One of the most commonly used approach is discrete event simulation (DES). This method models a process by primarily analyzing changes that occur due to certain events and their parameters. A process, in this context, refers to any sequence of actions of interest—for example, processing time for a component, raw material management transportation times, or even sales forecasting. In general, any event that can be mathematically parametrized can be included.
For example, in pharmaceutical manufacturing, disruptions in the supply chain can have a ripple effect on production schedules, regulatory compliance, and final product delivery. Imagine a delay in receiving a critical active ingredient – how does this impact batch processing? Will production lines remain idle, and if so, for how long? Can we use the time to utilize the equipment for another batch/recipe. How will this affect downstream operations, such as quality control and packaging? These and other questions find their answers with the DES. Complex pharmaceutical systems require careful consideration of cascading effects when disruptions occur. By modeling these scenarios, we can assess how resilient the system is, determine the cost of such disruptions, and explore alternative management strategies to mitigate risks and effectively utilize equipment.
DES models can be applied to a wide range of systems. Armed with this mathematical replica of a real-world process, they can study its behavior in different conditions and scenarios. A set of discrete events is defined and their impact on the overall system is evaluated. The impact can be measured, allowing for simulations of the system’s performance years into the future. This long-term perspective helps establish the financial constraints of the entire project and justifies the need for a specific investment.
The DES model provides users with a comprehensive view of their project’s potential. This mathematical model prioritizes transparency by presenting both best and worst-case scenarios – its likelihood and the timeframe in which it could occur. This empowers end-users to make informed decisions and set realistic expectations for their projects. Moreover, it gives the fundamentals when to start growing any technology and view optimum times for maintenance and service.
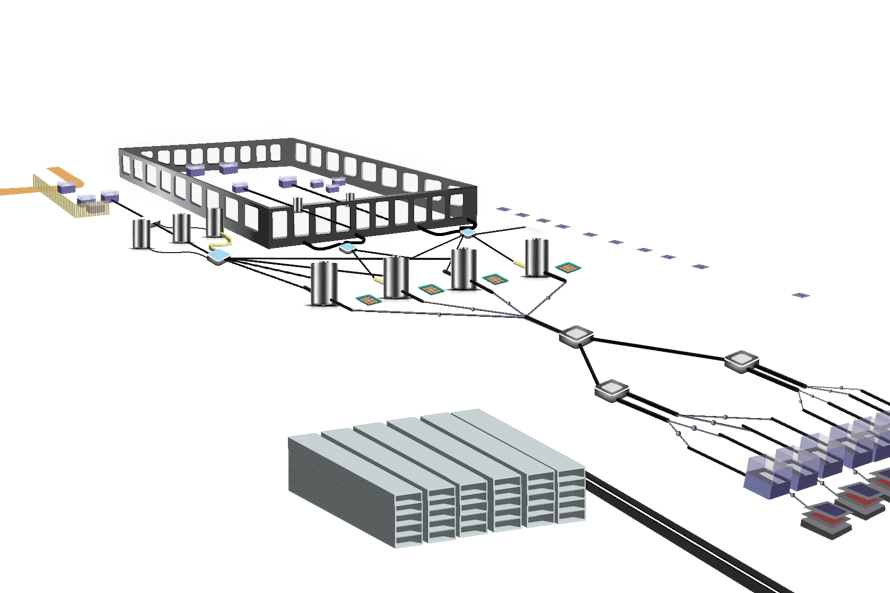
Sustaining Current Operations Success: DES as a Tool for Improvement
The second equally important aspect of the DES model, where significant value is added, is the optimization of existing systems. Many production facilities operate with machinery that has been in use for years, often functioning below their optimal efficiency. Optimization topic of interest in this respect can be:
- Determining the optimal speed of transport mechanisms – identifying the most efficient speed and capacity for a wide range of traditional transport systems, including roller, belt, AGV, and or any other.
- Maximizing machine utilization – analyze processing times for production units to achieve peak utilization. Additionally, evaluate how downtime for one or multiple machines impacts the overall production process and potential backup process/machine arrangements.
- Before launching full-scale production, process simulations generate Predictive Maintenance insights, allowing potential machine downtime to be anticipated and prevented. This ensures that no critical equipment unexpectedly enters maintenance mode, keeping operations smooth and deadlines intact.
- Optimizing logistics and storage capacity – determine the minimum required warehouse or logistics space to ensure uninterrupted production while avoiding excessive costs for unnecessary storage. On parallel we can adequately review any expected operational growths and include them in the overall design stages.
- Improving AGV fleet efficiency – as industries transition to Industry 4.0, more operations rely on industrial robots. Entire logistics centers are already operated by AGV (Automated Guided Vehicle) systems. We can determine the optimal number and parameters of AGVs, as well as simulate failure scenarios for one or multiple vehicles.
- Supporting continuous improvement (CI) efforts – adapting production to constant business changes requires ongoing process optimization. Through DES modeling, we can identify hidden patterns and process bottlenecks in not directly related operations and dynamically adjust the model to reflect evolving conditions. This allows for immediate corrective actions and ensures that CI is not just corporate jargon but a practical reality.
- Assessing human factors in production – despite technological advancements, many industries still rely heavily on manual labor. Evaluating the “human factor” in an operation is challenging. Through process simulation, we can establish time thresholds—even if we cannot quantify human variability exactly, we can measure its impact, for example optimal staffing levels required for a specific task or shift, moving beyond subjective assessments to data-driven decisions.
Final Takeaways: DES as a Key to Operational Resilience
Successful businesses must balance supply and demand, but with so many factors of influence, this is a complex challenge. А systematic approach can help identify this balance, enabling businesses to assess how real-world conditions affect their initial idea.
Rapid DES modeling reduces the need for high initial investments. Once the key parameters are defined, and the time and financial horizons are clear, detailed and adaptable simulation models can be developed. These models can be integrated with real production systems, allowing for real-time corrective actions when necessary.
By investing in DES modeling, companies gain predictability and resilience across their production, processes, machinery, and overall business operations.